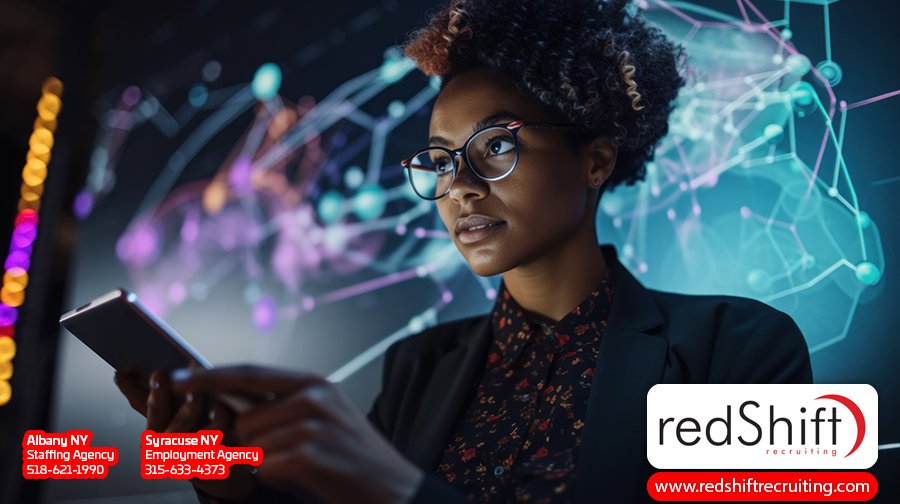
The Rise Of The Data Lakehouse: Definition, Advantages, And Use Cases
Introduction
Welcome to the world of data, where vast amounts of information flow like rivers and lakes. But in this ever-evolving landscape, a new player has emerged, ready to revolutionize how we store, manage, and analyze our data. Enter the data lakehouse.
Imagine a hybrid creature, born from the merging of two powerful entities: the scalability and flexibility of a data lake, and the structure and reliability of a full data warehouse system. This amalgamation gives birth to the data lakehouse – a unified platform that combines the best features of both worlds. It's like having an all-in-one solution that can handle any type or size of data while providing efficient storage, high performance analytics, and robust governance.
In this article, we will dive deep into the world of data lakehouses. We will explore their architecture, advantages, use cases, as well as real-world examples and benefits. We'll also discuss the challenges they present and consider future trends in this rapidly evolving field.
So whether you're an aspiring data scientist or a seasoned IT professional looking to stay ahead of the curve, join us on this exciting journey through the rise of the data lakehouse – where belonging to this cutting-edge community means harnessing the power of your data like never before.
Introduction to Data Lakehouse
What exactly is a data lakehouse and why is it becoming so popular in modern data management? Can it truly revolutionize the way organizations store, manage, and analyze their vast amounts of data?
Well, a data lakehouse can be defined as a unified platform that combines the best features of both a data warehouse and a data lake. It provides organizations with the ability to store and process large volumes of structured and unstructured data in its raw form, without needing to define a schema upfront. This flexibility allows for easy scalability and adaptability to changing business needs.
One of the key reasons why the rise of the data lakehouse is gaining traction is because it addresses some of the limitations of traditional approaches such as separate data warehouses or data lakes. Unlike data warehouses which require predefined schemas for ingesting structured data, or data lakes which lack proper governance for managing unstructured datasets, a data lakehouse offers an optimal solution by providing both structure and governance.
This means that organizations can now efficiently handle diverse types of information like text documents, images, videos, sensor readings, social media feeds, etc., all within one unified platform.
Moreover, by integrating structured and unstructured datasets into a single repository with strong governance policies in place, organizations can unlock new insights from their vast amount of stored information. Data scientists can now access raw datasets directly from the lakehouse architecture without having to go through complex ETL (Extract-Transform-Load) processes. This accelerates analytics workflows tremendously since machine learning models thrive on large volumes of diverse datasets.
The rise of the data lakehouse signifies an evolution in modern-day data management practices. By combining features from both traditional approaches like warehouses and lakes while addressing their limitations simultaneously, organizations gain more flexibility in storing various types of structured and unstructured datasets within one unified platform. This not only enables efficient data storage, but also empowers analysts and scientists to extract valuable insights from raw information swiftly.
Understanding the Data Lakehouse
Explore the transformative power of a data lakehouse, where you can effortlessly unlock the full potential of your data, seamlessly integrate and scale your operations, and experience simplified data management like never before.
With a data lakehouse, gone are the days of siloed data warehouses and fragmented data science workflows. Instead, you have a unified data platform that brings together raw data from various sources, enabling easier access and analysis. This convergence of data allows for improved business intelligence and decision-making capabilities.
In a data lakehouse architecture, raw data is stored in its original format without any predefined schema or structure. This flexibility enables organizations to capture vast amounts of diverse datasets rapidly. With direct access and the ability to store both structured and unstructured information in one place, businesses can leverage advanced analytics techniques to gain deeper insights into their operations.
The scalability aspect of a data lakehouse ensures that as your organization grows and generates more significant volumes of data, you can seamlessly expand your storage capacity without disrupting existing processes. Additionally, with simplified data management tools and practices like versioning, metadata tagging, and access controls built into the platform itself, managing large-scale datasets becomes much more efficient and cost-effective.
By harnessing the power of a unified data platform like a lakehouse architecture, organizations can truly capitalize on their valuable asset – their collected information – to drive innovation and stay ahead in today's competitive market.
Data Lakehouse Architecture
Discover the seamless integration of data lakes and warehouses in a powerful architecture that enables effortless data ingestion, processing, scalability, and performance optimization. The data lakehouse architecture combines the best features of both data lakes and warehouses to provide an efficient and flexible solution for managing large volumes of structured and unstructured data.
Data lakes are designed for storing raw, unprocessed data from various sources in its native format without any predefined schema or structure. On the other hand, most data warehouses now are optimized for querying structured data with predefined schemas. By integrating these two technologies, organizations can leverage the benefits of both worlds.
In a data lakehouse architecture, the raw data stored in the data lake architecture is processed using different frameworks such as Apache Spark or Apache Flink. These frameworks provide powerful tools for transforming and analyzing large datasets at scale. Data engineers can use these frameworks to perform complex ETL (Extract, Transform, Load) operations on the raw data to make it more suitable for analysis by business intelligence tools or machine learning algorithms.
Additionally, by leveraging the storage capabilities of a warehouse within the architecture, organizations can ensure that only high-quality processed data is available for consumption by data analysts and teams. This ensures that the insights derived from this architecture are accurate and reliable while maintaining strong control over access rights and permissions to protect sensitive information.
Advantages and Use Cases
Embrace the power of the data lakehouse architecture, where you can unlock the full potential of your diverse data types by seamlessly integrating storage, processing, and analytics into one dynamic platform that acts as a Swiss Army knife for your data needs.
With a data lakehouse, you no longer have to worry about separate systems for storing and analyzing your data. Traditional data warehouses often struggle with scalability and flexibility when it comes to handling diverse data types. However, with a data lakehouse, you can effortlessly handle structured, semi-structured, and unstructured data all in one place.
One major advantage of the data lakehouse architecture is its ability to support real-time analytics, machine learning, and business intelligence use cases. Whether you are analyzing large volumes of streaming sensor data or running complex machine learning algorithms on your datasets, the seamless integration of storage and processing capabilities in a data lakehouse enables faster insights and decision-making.
Data scientists alike benefit from this unified platform as they can easily access both raw and transformed datasets for their experiments. Additionally, cloud-based solutions like Azure Data Lake Storage provide low-cost object storage combined with high-performance SQL analysis capabilities, making it an ideal choice for big data workloads that require both cost-effectiveness and high performance.
The advantages offered by the rise of the data lakehouse make it an indispensable tool for organizations seeking scalable, flexible, cost-effective solutions for their diverse dataset needs.
Data Governance and Security
To ensure compliance, data quality, and data lineage in your organization, you must actively address the challenges of data security and access controls. With the rise of the data lakehouse architecture, which combines the best features of a data warehouse and a data lake, it becomes crucial to implement effective data governance and security measures.
The data lakehouse provides a centralized data management layer that allows you to enforce data quality standards across all your datasets. Data governance plays a vital role in maintaining the integrity of your data repository within the data lakehouse. By establishing clear policies and procedures for managing and protecting sensitive information, you can minimize the risk of unauthorized access or breaches.
Implementing robust access controls ensures that only authorized individuals can access certain datasets or perform specific actions on them. Additionally, incorporating security measures such as encryption and authentication protocols adds an extra layer of protection to your valuable datasets. By prioritizing data governance and security within your organization's data lakehouse infrastructure, you and data teams can ensure that you have reliable and actionable data at your fingertips while mitigating potential risks associated with unauthorized access or compromised integrity.
Real-World Examples and Benefits
One interesting statistic that highlights the benefits of implementing effective data governance and security measures in a data lakehouse is that organizations with strong data governance practices can expect a 20% increase in overall revenue. This demonstrates the significant impact that proper data management capabilities and protection of data can have on a business's bottom line. By ensuring that data is accurate, reliable, and secure within the data lakehouse, organizations can make more informed decisions and drive better business outcomes.
Real-world examples further illustrate the advantages of utilizing a data lakehouse. For instance, a large e-commerce company implemented a data lakehouse to combine their diverse datasets from various sources such as customer transactions, website interactions, and social media sentiment analysis. By leveraging the capabilities of the data lakehouse architecture, they were able to perform complex analytics and gain valuable insights into customer behavior patterns. This allowed them to optimize their marketing strategies, personalize customer experiences, and ultimately increase sales.
Another benefit of using a data lakehouse can be seen in healthcare organizations. With vast amounts of patient information stored across different systems like electronic health records (EHRs) and operational databases, it becomes crucial to integrate this fragmented data for meaningful analysis. By transforming these disparate sources into a unified format within the data lakehouse, healthcare providers can leverage advanced analytics to identify trends, improve diagnosis accuracy, and enhance patient care.
Implementing an effective data governance strategy coupled with robust security measures in a data lakehouse offers numerous benefits to organizations. Real-world examples highlight how businesses across various sectors have leveraged these advantages to drive revenue growth through improved decision-making capabilities and enhanced customer insights. Whether it's optimizing marketing strategies or enhancing patient care in healthcare settings, the use cases for leveraging the power of a well-managed data lakehouse are vast and impactful.
Challenges and Considerations
Addressing the challenges and considerations surrounding data integration, consistency, management practices, skills gap, organizational readiness, and change management is crucial for successful implementation and utilization of a well-managed enterprise data lakehouse. A data lakehouse combines the best features of both data warehouses and data lakes to provide a unified platform for storing and analyzing structured and unstructured data.
One of the main advantages of a data lakehouse is its ability to handle vast amounts of diverse data from multiple systems in real-time. However, this also poses challenges in terms of ensuring data integrity and consistency across all sources. Data integration becomes complex as different systems may have varying formats and structures, requiring careful mapping and transformation processes.
Another challenge is managing the storage and compute resources efficiently in a data lakehouse environment. While traditional data warehouses are optimized for structured querying using SQL with ACID transactions, a data lakehouse supports open-data management architectures that allow flexibility in processing various types of workloads including batch processing, stream processing, machine learning, and real-time analytics. This requires organizations to carefully consider their infrastructure requirements to ensure optimal performance while balancing costs.
Organizational readiness and change management are also key considerations when implementing a data lakehouse. The shift towards adopting new technologies like this can be disruptive to existing workflows and require upskilling or reskilling employees to make the most out of the platform's capabilities. It's essential for organizations to invest in training programs that enable employees to effectively use the tools available within the data lakehouse environment.
While there are numerous advantages associated with implementing a well-managed data lakehouse such as improved scalability, flexibility, cost-efficiency, and faster insights generation from diverse datasets; organizations must address various challenges related to integrating disparate sources with different formats while maintaining consistent quality standards across all datasets. Additionally, managing storage and compute resources effectively along with ensuring organizational readiness through proper change management practices are vital for successful data warehousing implementation. By overcoming these challenges and considering the necessary considerations, organizations can harness the full potential of a data lakehouse to derive valuable insights and gain a competitive edge in today's data-driven world.
Future Trends in Data Lakehouse
Amidst the rapidly evolving landscape of technology and cloud-native architectures, the future of data lakehouses is poised to transform with cutting-edge advancements and seamless integration possibilities.
As businesses continue to generate massive amounts of data from various sources, the demand for data lakehouses will only increase.
One of the key future trends in data lakehouses is their ability to access multiple systems and integrate with different platforms. This allows organizations to centralize their data in a single location while still being able to connect and extract insights from various sources storing data, such as databases, APIs, and streaming platforms.
Another future trend in data lakehouses is their potential for enabling advanced analytics and business intelligence. With a well-structured and organized data lakehouse, companies can leverage powerful analytical tools and algorithms to gain valuable insights into their operations, customer behavior, market trends, and more.
The integration of artificial intelligence (AI) technologies into data lakehouses opens up even greater possibilities for predictive analytics and machine learning applications.
Furthermore, as cloud object storage becomes increasingly popular due to its scalability and low cost, this technology will also play a significant role in shaping the future of data lakehouses.
Cloud object storage provides an efficient way to store vast amounts of unstructured or semi-structured data that can be easily accessed by multiple users or applications. By leveraging cloud object storage solutions within a data lakehouse architecture, organizations can achieve cost-effective scalability while ensuring high availability and durability of their data.
The future trends in data lakehouses offer numerous advantages for businesses seeking efficient ways to manage and analyze large volumes of diverse datasets.
The ability to access multiple systems, harness advanced analytics capabilities, integrate AI technologies, and leverage cloud object storage all contribute to making data lakehouses a powerful tool for driving informed decision-making processes across industries.
Whether it's optimizing operations or uncovering hidden patterns within vast datasets, embracing these future trends will undoubtedly unlock new opportunities for businesses aiming for a competitive advantage in the data-driven era.
Frequently Asked Questions
What are the key differences between a data lakehouse and a traditional data warehouse?
The key differences between a data lakehouse and a traditional data warehouse lie in their architecture, flexibility, and scalability.
Unlike a traditional data warehouse that follows a strict schema-on-write approach, a data lakehouse adopts a schema-on-read approach, allowing you to store raw and unstructured data as-is.
This means you can ingest and store vast amounts of diverse data types without the need for upfront transformation or modeling.
The data lakehouse also leverages modern cloud-based technologies like Apache Spark and Delta Lake, enabling you to perform advanced analytics and machine learning directly on the raw data.
Additionally, the scalability of a data lakehouse is unmatched as it can handle large volumes of structured and semi-structured data with ease.
With its ability to accommodate both batch and real-time data processing, the data lakehouse empowers organizations to derive valuable insights from their ever-growing datasets while maintaining agility in an evolving technological landscape.
By embracing this new paradigm, you can unlock the true potential of your data while keeping up with the demands of today's analytical requirements.
How does a data lakehouse handle data integration and transformation?
When it comes to data integration and transformation, a data lakehouse shines with its ability to handle vast amounts of diverse data in real-time.
With the rise of big data science machine learning, organizations are facing a staggering challenge - 80% of their time is spent on preparing and cleansing data for analysis.
However, a fascinating statistic reveals that by leveraging the power of a data lakehouse, companies can reduce this time-consuming process by up to 90%.
By storing raw and unprocessed data in its native format, a data lakehouse allows for seamless integration without the need for costly ETL processes.
This empowers analysts and data scientists to directly access and transform the information they need, making insights readily available at lightning speed.
Moreover, with advanced capabilities like schema-on-read and built-in common machine learning tools and algorithms, a data lakehouse provides an analytical playground where users can experiment and uncover valuable patterns effortlessly.
So if you're searching for an efficient way to handle your organization's integration and transformation needs while fostering collaboration among teams, embracing the power of a data lakehouse might be just what you need.
Can a data lakehouse support real-time analytics and streaming data?
Yes, a data lakehouse can support real-time analytics and streaming data. By combining the best of both worlds, a data lakehouse architecture allows you to store and process large volumes of structured and unstructured data in its raw form while also enabling real-time ingestion and analysis.
With its scalable infrastructure, the data lakehouse empowers you to capture and analyze streaming data as it arrives, providing timely insights for decision-making. Furthermore, the built-in capabilities for schema enforcement and indexing ensure efficient querying even in real-time scenarios.
By embracing a data lakehouse approach, you can harness the power of both batch processing and real-time analytics to gain valuable insights from your streaming data in a unified platform that fosters collaboration and drives innovation within your organization.
What are the common challenges in implementing and managing a data lakehouse?
What are the common challenges in implementing and managing a data lakehouse?
Well, let's delve into this intriguing topic. You see, when it comes to establishing and maintaining a data lakehouse, there are various hurdles that you may encounter along the way.
Have you ever wondered how to handle the vast amounts of diverse data that flow into your organization? Are you concerned about ensuring data quality and governance while balancing scalability and flexibility?
These challenges can be quite complex but fear not! With careful planning and strategic execution, you can overcome these obstacles and unlock the true potential of your data lakehouse.
So, let's explore together how you can navigate this fascinating journey towards efficient implementation and seamless management.
How does data governance work in a data lakehouse environment?
Data governance in a data lakehouse environment is crucial for ensuring the quality, integrity, and security of your data. It involves implementing policies, processes, and tools to define how data is collected, stored, accessed, and used within the lakehouse. By establishing clear guidelines and standards, you can effectively manage data assets, minimize risks associated with data breaches or compliance violations, and enable collaboration across teams.
With robust data governance practices in place, you can confidently unleash the power of your data lakehouse while maintaining control over your valuable information. So if you're looking to build a successful data lakehouse strategy that maximizes the potential of your data assets without compromising on security or compliance requirements, prioritizing strong data governance is key.
Conclusion
To fully leverage the potential of data lakehouses, it's essential for you to explore and embrace these future trends. They will undoubtedly revolutionize the way businesses manage and analyze their vast datasets.
With the definition of a data lakehouse being an open data management architecture that combines the best features of data lakes and data warehouses, it provides organizations with a unified platform for storing and processing both structured and unstructured data. This allows for greater flexibility in handling diverse data types from multiple sources, eliminating the need to maintain separate systems like traditional data warehouses.
By adopting a data lakehouse, you can benefit from its open file formats that enable seamless integration with existing cloud data lakes elsewhere. This means that your organization can easily migrate or extend your existing infrastructure without disrupting ongoing operations or losing valuable historical information.
The advantages of using a data lakehouse are numerous. First and foremost, it allows for simplified data management features even of complex datasets by providing a single source of truth. Instead of having fragmented data stored across various silos, a centralized repository enables efficient access and analysis.
Additionally, the ability to ingest multiple data sources into a single location ensures comprehensive visibility across all relevant information. This empowers organizations to gain deeper insights through holistic analytics that consider various aspects of their business operations. Moreover, utilizing advanced analytics tools on top of a data lakehouse facilitates real-time decision-making based on up-to-date information.
The rise of the data lakehouse presents exciting opportunities for businesses seeking efficient ways to manage and analyze their vast amounts of disparate datasets. By combining the benefits of both traditional lakes and data warehouses into one unified data architecture together, organizations can streamline their processes while gaining valuable insights from diverse sources.
Embracing this trend will not only enable you to stay ahead in an increasingly competitive market but also position your company as an industry leader in effective data management practices.