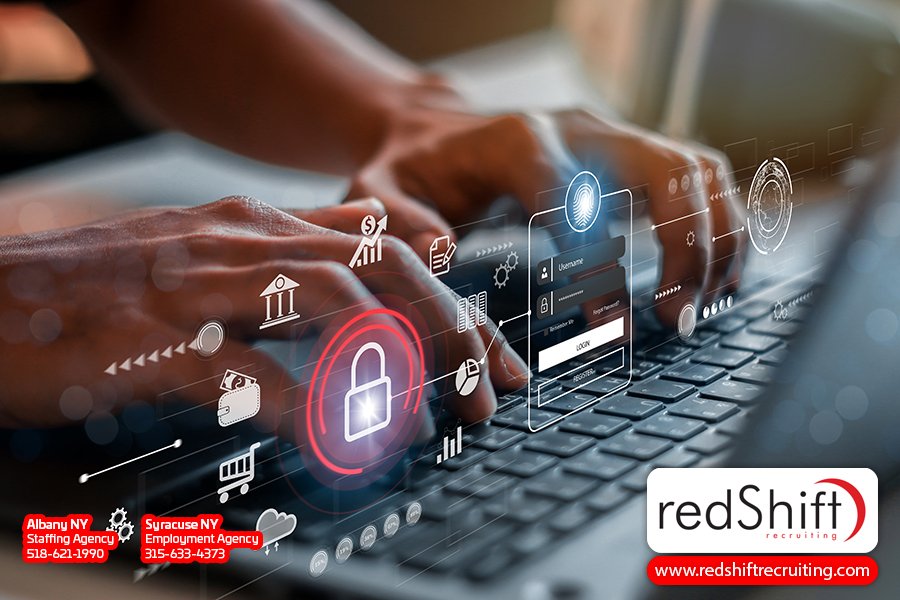
AI Security: The Threat Of Adversarial Attacks, Data Poisoning, and Model Bias
Introduction
In the world of AI and ML, where cutting-edge technology is constantly evolving, there exists a lurking threat that can undermine the very foundations of these powerful systems. Adversarial attacks, data poisoning, and model bias pose significant risks to the security and integrity of AI/ML models.
While these concepts may seem abstract and distant, they have the potential to impact our lives in ways we may not even realize.
Picture this: you're entrusting your personal information to an AI-powered application that promises convenience and efficiency. You feel a sense of belonging with this advanced technology, as if it understands your needs better than anyone else. But what if I told you that there are malicious actors out there who can manipulate this system? Adversarial attacks exploit vulnerabilities in AI/ML models by injecting deceptive inputs or perturbing existing ones to mislead the system's decision-making process. Your sense of belonging suddenly becomes fragile as you realize that even the most sophisticated algorithms can be tricked into making incorrect judgments.
Now, imagine another scenario: a seemingly harmless dataset used for training an ML model actually contains poisoned examples intentionally injected by someone with malicious intent. This act of data poisoning introduces biases into the model's learning process, leading it astray from its intended purpose. Suddenly, your trust in the accuracy and fairness of AI/ML systems starts wavering. The subconscious desire for belonging clashes with the realization that these systems can perpetuate inequality or discrimination due to biased training data.
In this article, we will delve into the threats posed by adversarial attacks, data poisoning, and model bias in AI/ML security. We will explore how these risks can compromise not only privacy but also societal values such as fairness and transparency. By understanding these challenges at a deeper level, we can begin to explore methods for mitigating their effects on our beloved AI/ML systems.
So buckle up because we are about to embark on a journey through the intricate world of AI/ML security, where the stakes are high, and the need for vigilance is paramount.
Introduction to AI/ML Security
In the world of AI/ML security, it's crucial to understand the threat posed by adversarial attacks, data poisoning, and model bias. As artificial intelligence (AI) systems become increasingly prevalent in various industries, ensuring their security has become a top priority.
AI and machine learning (ML) technologies have advanced significantly in recent years, revolutionizing the way we live and work. However, along with these advancements come many potential security threats and vulnerabilities that can be exploited by malicious actors.
Adversarial attacks are one such threat that AI/ML systems face. These attacks involve manipulating input data in a way that can deceive the system into producing incorrect or undesired outputs. Adversaries exploit vulnerabilities in the algorithms or models themselves to trick the system into making wrong decisions or causing harm.
Data poisoning is another significant concern for AI security. It involves injecting malicious data into training datasets with the intention of compromising the performance of ML models during training or inference stages. By tampering with the training data, attackers can manipulate model behavior and potentially cause severe consequences.
Model bias is yet another aspect of AI/ML security that cannot be overlooked. Models trained on biased datasets may exhibit discriminatory behavior when making decisions or predictions. This bias can be unintentionally introduced through biased training data or even inadvertently learned from societal biases present in human-labeled datasets.
Addressing this issue requires careful analysis and validation of datasets to ensure fairness and prevent any discriminatory outcomes.
To combat these threats effectively, organizations must implement robust security processes and operations specific to AI systems. Security analysts need to continuously monitor security processes for potential vulnerabilities and develop appropriate countermeasures using specialized security tools designed for protecting AI/ML models against adversarial attacks, data poisoning, and model bias.
By taking proactive measures to improve AI/ML security practices, we can mitigate risks associated with these emerging technologies and ensure their safe deployment across various domains, such as healthcare, finance, autonomous vehicles, artificial intelligence, and cybersecurity. Implementing robust security tools and strategies can help organizations safeguard their AI/ML models from malicious attacks, ensure the integrity of data used for training, and mitigate biases that may result in unfair outcomes.
This proactive approach is vital to building trust in AI/ML systems and promoting their responsible adoption in critical applications.
Adversarial Attacks
Contrarily, it's alarming to realize that even our most advanced AI technologies can be easily manipulated through adversarial attacks.
These attacks exploit vulnerabilities in deep learning techniques and machine learning algorithms, allowing malicious actors to manipulate the behavior of AI systems for their own gain.
One real-world example of this is the manipulation of autonomous cars through altered stop signs.
In a study conducted by researchers at the University of Washington, they were able to successfully alter stop signs in a way that confused autonomous cars.
By placing stickers strategically on the stop signs, they were able to fool the AI systems into misclassifying them as other objects, such as speed limits or yield signs.
The shocking result was a staggering 90% success rate in confusing these autonomous vehicles.
This demonstrates how adversarial attacks can pose a significant threat to the security and reliability of AI-powered systems.
To address this issue, organizations need to employ robust threat intelligence and have dedicated security teams focused on monitoring threat detection and mitigating adversarial attacks.
Cybersecurity solutions specifically designed for detecting and preventing such attacks should also be implemented.
Additionally, model bias needs to be taken into consideration when developing AI models.
Bias can lead to skewed results and make models more vulnerable to adversarial attacks.
Therefore, it's crucial for developers and researchers to continuously evaluate their models for potential biases and take steps towards minimizing them.
Overall, it's imperative that we recognize the potential risks associated with adversarial attacks in AI/ML systems.
By understanding these threats and implementing appropriate security measures, we can ensure the security operations integrity and reliability of our AI technologies in an increasingly interconnected world.
Data Poisoning
To fully appreciate the extent of potential manipulation in AI systems, you'll be captivated by the concept of data poisoning and how it can compromise the reliability of your machine learning algorithms and models.
Data poisoning refers to the injection of malicious data into a system's training set with the intention to deceive or manipulate AI-powered systems. This form of attack aims to corrupt the machine learning and process, causing the model to make incorrect predictions or exhibit biased behaviors.
Data poisoning attacks can have severe consequences for organizations relying on AI/ML technologies. Here are some key points to consider:
Compromising cyber defense: By injecting poisoned data into an organization's training dataset, attackers can exploit vulnerabilities in AI systems and bypass security measures. This allows them to gain unauthorized access, exfiltrate sensitive information, or launch further attacks.
Challenging incident response: Data poisoning attacks pose significant challenges for incident response teams. Traditional security alerts might not detect these attacks since they target the integrity of machine learning models rather than traditional security vulnerabilities.
Mitigating vulnerabilities: To effectively defend against data poisoning, organizations need robust threat detection and mitigation strategies tailored specifically for AI-powered systems. Security teams must continuously monitor their datasets for anomalies and apply techniques such as outlier detection and anomaly analysis to identify potential instances of data poisoning.
By understanding the implications of data poisoning and its impact on incident response to artificial intelligence (AI) security incidents, organizations can develop proactive measures that strengthen their defenses against adversarial attacks targeting machine learning models.
Model Bias
Alright folks, let's dive into the fascinating world of model bias and how it can impact our beloved AI systems. Model bias refers to the systematic error or unfairness that's embedded in AI models, leading to discriminatory outcomes. This bias can arise from various sources such as biased training data, biased labels, or even biased algorithms. It's essential to understand and address model bias because it can have far-reaching consequences in domains like facial recognition systems.
One example of model bias is racial or gender bias in facial recognition systems. These systems are trained on large datasets that may not be diverse enough, resulting in inaccurate identification or misidentification of certain racial or gender groups. For instance, studies have shown that facial recognition algorithms have higher error rates for women and people with darker skin tones compared to men and those with lighter skin tones. This bias can lead to negative impacts, including unjust surveillance practices, false criminal identifications, and a reinforcement of societal biases.
To mitigate this issue, researchers are focusing on developing more diverse training datasets and incorporating fairness metrics into the evaluation process for AI models. By addressing model bias head-on, we can improve the fairness and effectiveness of AI systems while ensuring equal treatment for all individuals regardless of their race or gender.
Model bias poses a significant challenge to the field of AI/ML security. It necessitates a comprehensive approach involving deep learning techniques, robust security postures, and effective endpoint security measures. Understanding different attack strategies used by adversaries is crucial in building resilient AI analysis frameworks that account for potential biases introduced during training processes. Additionally, organizations should invest in developing a strong cybersecurity workforce equipped with skills necessary to identify and combat model biases effectively.
By taking these steps proactively, we can create a safer and more inclusive environment where AI technologies serve everyone equally without perpetuating discrimination or unfairness based on race or gender.
Mitigating Risks in AI/ML
However, it's crucial to address the risks involved in AI/ML systems to ensure a safer and more trustworthy future.
Mitigating these risks requires a multi-faceted approach that encompasses various strategies and techniques.
One aspect of mitigating risks in AI/ML is through effective cybersecurity measures. Cybersecurity teams play a vital role in identifying and remediating threats posed by adversarial attacks, data poisoning, and model bias.
To effectively mitigate risks in AI/ML systems, cybersecurity teams need to be equipped with advanced tools and technologies. False positives are a common challenge faced by these security teams, when dealing with security incidents. Leveraging natural language processing algorithms can help improve the accuracy of threat detection and reduce false alarms. Additionally, risk prediction models can assist cybersecurity teams in proactively identifying potential vulnerabilities or attack vectors before they're exploited.
Another critical aspect of mitigating risks involves addressing model bias. Model biases occur when datasets used for training favor certain demographics or exhibit discriminatory patterns. To overcome this challenge, diverse datasets that include representative samples from different groups must be used during model development. Additionally, ongoing monitoring and evaluation of models can help identify any biases introduced during the training process.
Moreover, efforts should be made to actively remediate threats caused by malicious files or data artifacts that may compromise system security. Continuous monitoring of system behavior can aid in detecting any suspicious activities or anomalous patterns indicative of an attack or tampering attempt.
By implementing robust cybersecurity measures and adopting strategies to minimize model bias, organizations can enhance the own security posture and trustworthiness of their AI/ML systems. This not only safeguards sensitive information but also ensures fair treatment across different user groups while promoting inclusivity within technology-driven solutions.
Frequently Asked Questions
How can AI/ML models be protected from adversarial attacks?
To protect AI/ML models from adversarial attacks, you need to employ a multi-layered cyber defense strategy.
First and foremost, you must fortify the model by implementing robust security measures that can withstand even the most sophisticated attacks. This involves carefully monitoring and analyzing incoming data to identify potential threats and mitigate vulnerabilities beforehand.
Additionally, incorporating techniques like input sanitization and anomaly detection can help detect and mitigate adversarial inputs.
Furthermore, regular updates and patches should be applied to address any known vulnerabilities in the underlying software or framework used for training the model.
It's also crucial to establish strict access controls and authentication mechanisms to prevent unauthorized access to sensitive data or code.
Lastly, conducting comprehensive penetration testing exercises can help uncover any weaknesses in the system's defenses before they are exploited by attackers.
Remember, protecting your AI/ML models requires an unwavering commitment to security that goes beyond simply relying on standard precautions. It necessitates a hyper-vigilant approach and security team that leaves no stone unturned in safeguarding your valuable assets from malicious actors seeking to exploit them for their own gain.
What are the common techniques used in data poisoning attacks?
Common techniques used in data poisoning attacks include the injection of malicious data into the training dataset, manipulation of feature values, and exploiting vulnerabilities in the data preprocessing stage.
In these attacks, adversaries aim to introduce subtle changes to the training data that can lead to biased or inaccurate model predictions. They may strategically modify a small subset of training instances or even corrupt a significant portion of the dataset to manipulate the deep learning the process.
Adversaries often leverage knowledge about the target model's architecture and algorithms to craft their attack strategies effectively. By understanding how models are trained and making strategic alterations to the input data, attackers can exploit vulnerabilities in AI/ML systems, compromising their integrity and performance.
It is crucial for researchers and practitioners alike to be aware of these common techniques so that they can develop robust defenses against such data poisoning attacks.
How does model bias affect the performance and fairness of AI/ML systems?
Model bias can significantly impact the performance and fairness of AI/ML systems. It occurs when the trained model exhibits a systematic error or prejudice in its predictions. This bias can arise from various sources, such as biased training data or biased algorithm design. When a model is biased, it can lead to discriminatory outcomes, favoring certain groups over others or perpetuating existing societal biases.
This not only hampers the accuracy and effectiveness of AI/ML systems but also raises ethical concerns about fairness and equity. To address this issue, researchers are actively working on developing techniques to detect and mitigate model bias, with the ultimate goal of creating more inclusive and unbiased AI/ML systems that benefit all individuals equally.
Are there any specific industries or applications that are more vulnerable to adversarial attacks?
In the realm of adversarial attacks, certain industries and applications are more susceptible to these malicious tactics.
One such vulnerable field is autonomous vehicles, where attackers can manipulate sensor data or visual cues to deceive the system into misinterpreting its surroundings. This puts not only the passengers at risk but also poses a significant threat to public safety.
Similarly, in financial services, adversarial attacks can be especially detrimental as fraudsters may exploit vulnerabilities in AI models to bypass security measures and gain unauthorized access to sensitive information or perform illicit transactions.
Furthermore, industries heavily reliant on image recognition systems like healthcare and defense may face targeted adversarial attacks aimed at manipulating medical images or deceiving surveillance systems.
It is crucial for organizations operating in these sectors to prioritize AI/ML security measures and stay vigilant against ever-evolving threats posed by adversaries seeking to exploit their vulnerabilities. By using threat intelligence and fostering a proactive approach towards securing AI systems, these industries can mitigate the risks associated with adversarial attacks and ensure the integrity and trustworthiness of their technology solutions.
What are the potential consequences of not mitigating the risks associated with AI/ML security threats?
Not mitigating the risks associated with AI/ML security threats can have severe consequences. Adversarial attacks, data poisoning, and model bias can all lead to significant damage in various industries and applications.
Without taking action to address these threats, your organization may suffer from compromised systems, loss of sensitive data, and reputational damage. Adversarial attacks can manipulate AI models, leading to incorrect decisions or malicious behavior.
Data poisoning can introduce biased or corrupted data into your training datasets, resulting in inaccurate predictions and unreliable outcomes. Model bias can further exacerbate this issue by perpetuating unfair or discriminatory practices.
Failing to mitigate these risks not only undermines the integrity and effectiveness of your AI/ML systems but also poses a threat to privacy, fairness, and trust in your organization's capabilities.
By proactively implementing robust security measures and continuously monitoring for potential vulnerabilities, you can safeguard against these consequences and ensure the responsible use of AI/ML technologies in your industry or application.
Conclusion
In conclusion, you now have the power to shape a safer and more trustworthy future for AI/ML systems by actively mitigating risks and ensuring fairness in technology-driven solutions.
By understanding the threats of adversarial attacks, data poisoning, and model bias, you can make informed decisions to protect your AI/ML models from potential vulnerabilities.
Stay ahead of the game by continuously monitoring and updating your systems with the latest in security tools and measures.
Drawing conclusions from the background information provided, it's evident that taking action to mitigate risks in AI/ML is crucial.
With high-speed advancements in technology, it's essential to prioritize security and fairness.
Incorporating more data into training sets can help identify patterns and potential vulnerabilities.
Additionally, leveraging prescriptive insights can provide valuable guidance for making critical decisions when it comes to securing AI/ML models.
Remember, staying proactive rather than reactive is key when it comes to maintaining a secure environment for AI/ML systems.
By following these guidelines and actively addressing potential risks, you can contribute to creating a safer future where trust in AI/ML technology remains intact.